AI for Radiology
Posted on Wed 25 September 2024 in research
The application of Artificial Intelligence (AI) in radiology has numerous benefits, including improved diagnostic accuracy for X-ray and CT scans, enhanced image analysis of MRI and PET scans, and increased efficiency in reviewing ultrasound and mammography images. AI-powered systems can automate routine tasks related to chest X-rays, abdominal CTs, and bone density scans, freeing up radiologists to focus on complex cases like brain MRIs, cardiac CTA scans, and breast cancer screening mammograms. For example, AI can be used to detect active pulmonary tuberculosis (TB) by analyzing X-ray images for signs of disease progression, such as cavitation or fibrosis. By automating the detection of these changes, AI-powered systems can help healthcare professionals diagnose TB, ultimately improving patient outcomes.
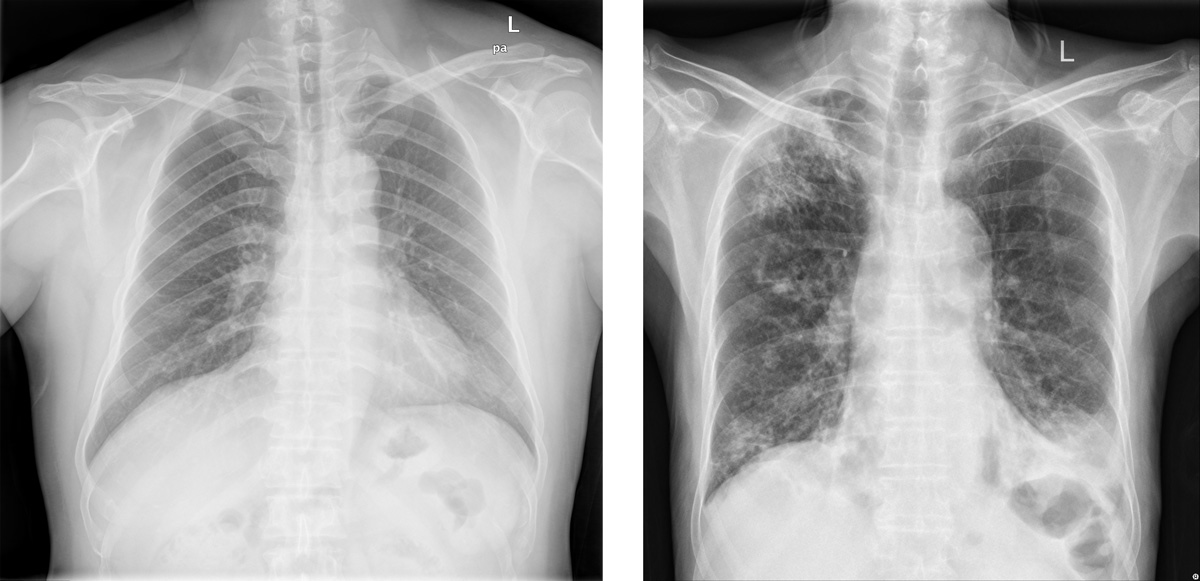
Example Application: Radiological signs on healthy (left) and active Pulmonary TB-affected lungs (right). AI models can be used to detect TB from CXR images.
Partnerships
- Prof. Dr. Med. Anete Trajman, Dr. José Manoel de Seixas, Dr. Natanael Moura Jr., from the Federal University of Rio de Janeiro
- Prof. Dr. Med. Lucia Mazzolai, from CHUV (Lausanne university hospital), Switzerland
- Prof. Manuel Günther, University of Zürich, Switzerland
- Dr. Eugenio Canes and André Baceti, Murabei Data Science, Brazil
Radiomics: In [1] We've developed an evaluation framework for extracting 3D deep radiomics features using pre-trained neural networks on real computed tomography (CT) scans, allowing comprehensive quantification of tissue characteristics. We compared these new features with standard hand-crafted radiomic features, demonstrating that our proposed 3D deep learning radiomics are at least twice more stable across different CT parameter variations than any category of traditional features. Notably, even when trained on an external dataset and task, our generic deep radiomics showed excellent stability and discriminative power for tissue characterization between different classes of liver lesions and normal tissue, with an average accuracy of 93.5%.
Chest X-ray CAD for Tuberculosis: In [2], We conducted a review of computer-aided detection (CAD) software for TB detection, highlighting its potential as a valuable tool but also several implementation challenges. Our assessment emphasizes the need for further research to address issues related to diagnostic heterogeneity, regulatory frameworks, and technology adaptation to meet the needs of high-burden settings and vulnerable populations. In [3] we proposed an new approach to develop more generalizable computer-aided detection (CAD) systems for pulmonary tuberculosis (PT) screening from Chest X-Ray images. Our method used radiological signs as intermediary proxies to detect PT, rather than relying on direct image-to-probability detection techniques that often fail to generalize across different datasets. We developed a multi-class deep learning model that maps images to 14 key radiological signs, followed by a second model that predicts PT diagnosis from these signs. Our approach demonstrated superior generalization capabilities compared to traditional CAD models, achieving higher area under the specificity vs. sensitivity curve (AUC) values on cross-dataset evaluation scenarios. Building on this, in [4] we developed reliable techniques to train deep learning models for PT detection. By pre-training a neural network on a proxy task and using a technique called Mixed Objective Optimization Network (MOON) to balance classes during training, we demonstrate that our approach can improve alignment with human experts' decision-making processes while maintaining perfect classification accuracy. Notably, this method also enhances generalization on unseen datasets, making it more suitable for real-world applications. We made our source code publicly available online for reproducibility purposes.
Semanatic Segmentation: In [5] we investigated the impact of digitization on X-Ray images and their effect on deep neural networks trained for lung segmentation, highlighting the need to adapt these models to accurately analyze digitized data. Our results show that while our model performs exceptionally well (AUPRC: 0.99) at identifying lung regions in digital X-Rays, its performance drops significantly (AUPRC: 0.90) when applied to digitized images. We also found that traditional performance metrics, such as maximum F1 score and area under the precision-recall curve (AUPRC), may not be sufficient to characterize segmentation problems in test images, particularly due to the natural connectivity of lungs in X-Ray images.
Demographic Fairness: In [6] we tackled the challenge of ensuring consistent performance and fairness in machine learning models for medical image diagnostics, with a focus on chest X-ray images. We proposed using Foundation Models as an embedding extractor to create groups representing protected attributes, such as gender and age. This approach can effectively group individuals by gender in both in- and out-of-distribution scenarios, reducing bias by up to 6.2%. However, the model's robustness in handling age attributes is limited, highlighting a need for more fundamentally fair and robust Foundation models. These findings contribute to the development of more equitable medical diagnostics, particularly where protected attribute information is lacking.
Bibliography
[1] Oscar Jimenez-del-Toro, Christoph Aberle, Roger Schaer, Michael Bach, Kyriakos Flouris, Ender Konukoglu, Bram Stieltjes, Markus M. Obmann, André Anjos, Henning Müller, and Adrien Depeursinge. Comparing stability and discriminatory power of hand-crafted versus deep radiomics: a 3d-printed anthropomorphic phantom study. In Proceedings of the 12th European Workshop on Visual Information Processing. September 2024.
@inproceedings{euvip-2024-2,
author = {Jimenez-del-Toro, Oscar and Aberle, Christoph and Schaer, Roger and Bach, Michael and Flouris, Kyriakos and Konukoglu, Ender and Stieltjes, Bram and Obmann, Markus M. and Anjos, Andr{\'{e}} and M{\"{u}}ller, Henning and Depeursinge, Adrien},
month = "September",
title = "Comparing Stability and Discriminatory Power of Hand-crafted Versus Deep Radiomics: A 3D-Printed Anthropomorphic Phantom Study",
booktitle = "Proceedings of the 12th European Workshop on Visual Information Processing",
year = "2024",
abstract = "Radiomics have the ability to comprehensively quantify human tissue characteristics in medical imaging studies. However, standard radiomic features are highly unstable due to their sensitivity to scanner and reconstruction settings. We present an evaluation framework for the extraction of 3D deep radiomics features using a pre-trained neural network on real computed tomography (CT) scans for tissue characterization. We compare both the stability and discriminative power of the proposed 3D deep learning radiomic features versus standard hand-crafted radiomic features using 8 image acquisition protocols with a 3D-printed anthropomorphic phantom containing 4 classes of liver lesions and normal tissue. Even when the deep learning model was trained on an external dataset and for a different tissue characterization task, the resulting generic deep radiomics are at least twice more stable on 8 CT parameter variations than any category of hand-crafted features. Moreover, the 3D deep radiomics were also discriminative for the tissue characterization between 4 classes of liver tissue and lesions, with an average discriminative power of 93.5\\%.",
pdf = "https://publications.idiap.ch/attachments/papers/2024/Jimenez-del-Toro\_EUVIP2024\_2024.pdf"
}
[2] C. Geric, Z. Z. Qin, C. M. Denkinger, S. V. Kik, B. Marais, André Anjos, P.-M. David, F. A. Khan, and A. Trajman. The rise of artificial intelligence reading of chest x-rays for enhanced tb diagnosis and elimination. INT J TUBERC LUNG DIS, May 2023. doi:10.5588/ijtld.22.0687.
@article{ijtld-2023,
author = "Geric, C. and Qin, Z. Z. and Denkinger, C. M. and Kik, S. V. and Marais, B. and Anjos, André and David, P.-M. and Khan, F. A. and Trajman, A.",
title = "The rise of artificial intelligence reading of chest X-rays for enhanced TB diagnosis and elimination",
doi = "10.5588/ijtld.22.0687",
abstract = "We provide an overview of the latest evidence on computer-aided detection (CAD) software for automated interpretation of chest radiographs (CXRs) for TB detection. CAD is a useful tool that can assist in rapid and consistent CXR interpretation for TB. CAD can achieve high sensitivity TB detection among people seeking care with symptoms of TB and in population-based screening, has accuracy on-par with human readers. However, implementation challenges remain. Due to diagnostic heterogeneity between settings and sub-populations, users need to select threshold scores rather than use pre-specified ones, but some sites may lack the resources and data to do so. Efficient standardisation is further complicated by frequent updates and new CAD versions, which also challenges implementation and comparison. CAD has not been validated for TB diagnosis in children and its accuracy for identifying non-TB abnormalities remains to be evaluated. A number of economic and political issues also remain to be addressed through regulation for CAD to avoid furthering health inequities. Although CAD-based CXR analysis has proven remarkably accurate for TB detection in adults, the above issues need to be addressed to ensure that the technology meets the needs of high-burden settings and vulnerable sub-populations.",
journal = "INT J TUBERC LUNG DIS",
volume = "27",
journaltitle = "International Journal of Tuberculosis and Lung Diseases",
year = "2023",
month = "May",
keywords = "computer-aided detection; chest radiology; pulmonary disease; tuberculosis; AI technology"
}
[3] Geoffrey Raposo, Anete Trajman, and André Anjos. Pulmonary tuberculosis screening from radiological signs on chest x-ray images using deep models. In Union World Conference on Lung Health. The Union, November 2022.
@inproceedings{union-2022,
author = "Raposo, Geoffrey and Trajman, Anete and Anjos, Andr{\'{e}}",
month = "November",
title = "Pulmonary Tuberculosis Screening from Radiological Signs on Chest X-Ray Images Using Deep Models",
booktitle = "Union World Conference on Lung Health",
year = "2022",
addendum = "(Issued from master thesis supervision)",
date = "2022-11-01",
organization = "The Union",
abstract = "Background: The World Health Organization has recently recommended the use of computer-aided detection (CAD) systems for screening pulmonary tuberculosis (PT) in Chest X-Ray images. Previous CAD models are based on direct image to probability detection techniques - and do not generalize well (from training to validation databases). We propose a method that overcomes these limitations by using radiological signs as intermediary proxies for PT detection. Design/Methods: We developed a multi-class deep learning model, mapping images to 14 radiological signs such as cavities, infiltration, nodules, and fibrosis, using the National Institute of Health (NIH) CXR14 dataset, which contains 112,120 images. Using three public PTB datasets (Montgomery County - MC, Shenzen - CH, and Indian - IN), summing up 955 images, we developed a second model mapping F probabilities to PTB diagnosis (binary labels). We evaluated this approach for its generalization capabilities against direct models, learnt directly from PTB training data or by transfer learning via cross-folding and cross-database experiments. The area under the specificity vs. sensitivity curve (AUC) considering all folds was used to summarize the performance of each approach. Results: The AUC for intra-dataset tests baseline direct detection deep models achieved 0.95 (MC), 0.95 (CH) and 0.91 (IN), with up to 35\\% performance drop on a cross-dataset evaluation scenario. Our proposed approach achieved AUC of 0.97 (MC), 0.90 (CH), and 0.93 (IN), with at most 11\\% performance drop on a cross-dataset evaluation (Table/figures). In most tests, the difference was less than 5\\%. Conclusions: A two-step CAD model based on radiological signs offers an adequate base for the development of PT screening systems and is more generalizable than a direct model. Unlike commercially available CADS, our model is completely reproducible and available open source at https://pypi.org/project/bob.med.tb/."
}
[4] Özgür Güler, Manuel Günther, and André Anjos. Refining tuberculosis detection in cxr imaging: addressing bias in deep neural networks via interpretability. In Proceedings of the 12th European Workshop on Visual Information Processing. September 2024.
@inproceedings{euvip-2024-1,
author = {G{\"{u}}ler, {\"{O}}zg{\"{u}}r and G{\"{u}}nther, Manuel and Anjos, Andr{\'{e}}},
month = "September",
title = "Refining Tuberculosis Detection in CXR Imaging: Addressing Bias in Deep Neural Networks via Interpretability",
booktitle = "Proceedings of the 12th European Workshop on Visual Information Processing",
year = "2024",
abstract = "Automatic classification of active tuberculosis from chest X-ray images has the potential to save lives, especially in low- and mid-income countries where skilled human experts can be scarce. Given the lack of available labeled data to train such systems and the unbalanced nature of publicly available datasets, we argue that the reliability of deep learning models is limited, even if they can be shown to obtain perfect classification accuracy on the test data. One way of evaluating the reliability of such systems is to ensure that models use the same regions of input images for predictions as medical experts would. In this paper, we show that pre-training a deep neural network on a large-scale proxy task, as well as using mixed objective optimization network (MOON), a technique to balance different classes during pre-training and fine-tuning, can improve the alignment of decision foundations between models and experts, as compared to a model directly trained on the target dataset. At the same time, these approaches keep perfect classification accuracy according to the area under the receiver operating characteristic curve (AUROC) on the test set, and improve generalization on an independent, unseen dataset. For the purpose of reproducibility, our source code is made available online.",
pdf = "https://publications.idiap.ch/attachments/papers/2024/Guler\_EUVIP24\_2024.pdf"
}
[5] Matheus A. Renzo, Natália Fernandez, André A. Baceti, Natanael Nunes Moura Junior, and André Anjos. Development of a lung segmentation algorithm for analog imaged chest x-ray: preliminary results. In Anais do 15. Congresso Brasileiro de Inteligência Computacional, 1–8. SBIC, October 2021. URL: https://sbic.org.br/eventos/cbic_2021/cbic2021-123/, doi:10.21528/CBIC2021-123.
@inproceedings{cbic-2021,
author = "Renzo, Matheus A. and Fernandez, Natália and Baceti, André A. and Moura Junior, Natanael Nunes and Anjos, André",
title = "Development of a lung segmentation algorithm for analog imaged chest X-Ray: preliminary results",
url = "https://sbic.org.br/eventos/cbic\_2021/cbic2021-123/",
pdf = "https://www.idiap.ch/\textasciitilde aanjos/papers/cbic-2021.pdf",
doi = "10.21528/CBIC2021-123",
shorttitle = "Development of a lung segmentation algorithm for analog imaged chest X-Ray",
addendum = "(Issued from internship supervision)",
abstract = "Analog X-Ray radiography is still used in many underdeveloped regions around the world. To allow these populations to benefit from advances in automatic computer-aided detection (CAD) systems, X-Ray films must be digitized. Unfortunately, this procedure may introduce imaging artefacts, which may severely impair the performance of such systems. This work investigates the impact digitized images may cause to deep neural networks trained for lung (semantic) segmentation on digital x-ray samples. While three public datasets for lung segmentation evaluation exist for digital samples, none are available for digitized data. To this end, a U-Net-style architecture was trained on publicly available data, and used to predict lung segmentation on a newly annotated set of digitized images. Using typical performance metrics such as the area under the precision-recall curve (AUPRC), our results show that the model is capable to identify lung regions at digital X-Rays with a high intra-dataset (AUPRC: 0.99), and cross-dataset (AUPRC: 0.99) efficiency on unseen test data. When challenged against digitized data, the performance is substantially degraded (AUPRC: 0.90). Our analysis also suggests that typical performance markers, maximum F1 score and AUPRC, seems not to be informative to characterize segmentation problems in test images. For this goal pixels does not have independence due to natural connectivity of lungs in images, this implies that a lung pixel tends to be surrounded by other lung pixels. This work is reproducible. Source code, evaluation protocols and baseline results are available at: https://pypi.org/project/bob.ip.binseg/.",
eventtitle = "Congresso Brasileiro de Inteligência Computacional",
pages = "1--8",
booktitle = "Anais do 15. Congresso Brasileiro de Inteligência Computacional",
year = "2021",
month = "October",
publisher = "{SBIC}"
}
[6] Dilermando Queiroz Neto, André Anjos, and Lilian Berton. Using backbone foundation model for evaluating fairness in chest radiography without demographic data. In Proceedings of the International Conference on Medical Image Computing and Computer Assisted Intervention (MICCAI). October 2024.
@inproceedings{miccai-2024,
author = "Queiroz Neto, Dilermando and Anjos, Andr{\'{e}} and Berton, Lilian",
keywords = "Fairness, Foundation Model, Medical Image",
month = "October",
title = "Using Backbone Foundation Model for Evaluating Fairness in Chest Radiography Without Demographic Data",
booktitle = "Proceedings of the International Conference on Medical Image Computing and Computer Assisted Intervention (MICCAI)",
year = "2024",
abstract = "Ensuring consistent performance across diverse populations and incorporating fairness into machine learning models are crucial for advancing medical image diagnostics and promoting equitable healthcare. However, many databases do not provide protected attributes or contain unbalanced representations of demographic groups, complicating the evaluation of model performance across different demographics and the application of bias mitigation techniques that rely on these attributes. This study aims to investigate the effectiveness of using the backbone of Foundation Models as an embedding extractor for creating groups that represent protected attributes, such as gender and age. We propose utilizing these groups in different stages of bias mitigation, including pre-processing, in-processing, and evaluation. Using databases in and out-of-distribution scenarios, it is possible to identify that the method can create groups that represent gender in both databases and reduce in 4.44\\% the difference between the gender attribute in-distribution and 6.16\\% in out-of-distribution. However, the model lacks robustness in handling age attributes, underscoring the need for more fundamentally fair and robust Foundation models. These findings suggest a role in promoting fairness assessment in scenarios where we lack knowledge of attributes, contributing to the development of more equitable medical diagnostics.",
pdf = "https://publications.idiap.ch/attachments/papers/2024/QueirozNeto\_CVPR\_2024.pdf"
}