Computer Vision and Deep Learning for Biometrics
Posted on Tue 01 May 2018 in research
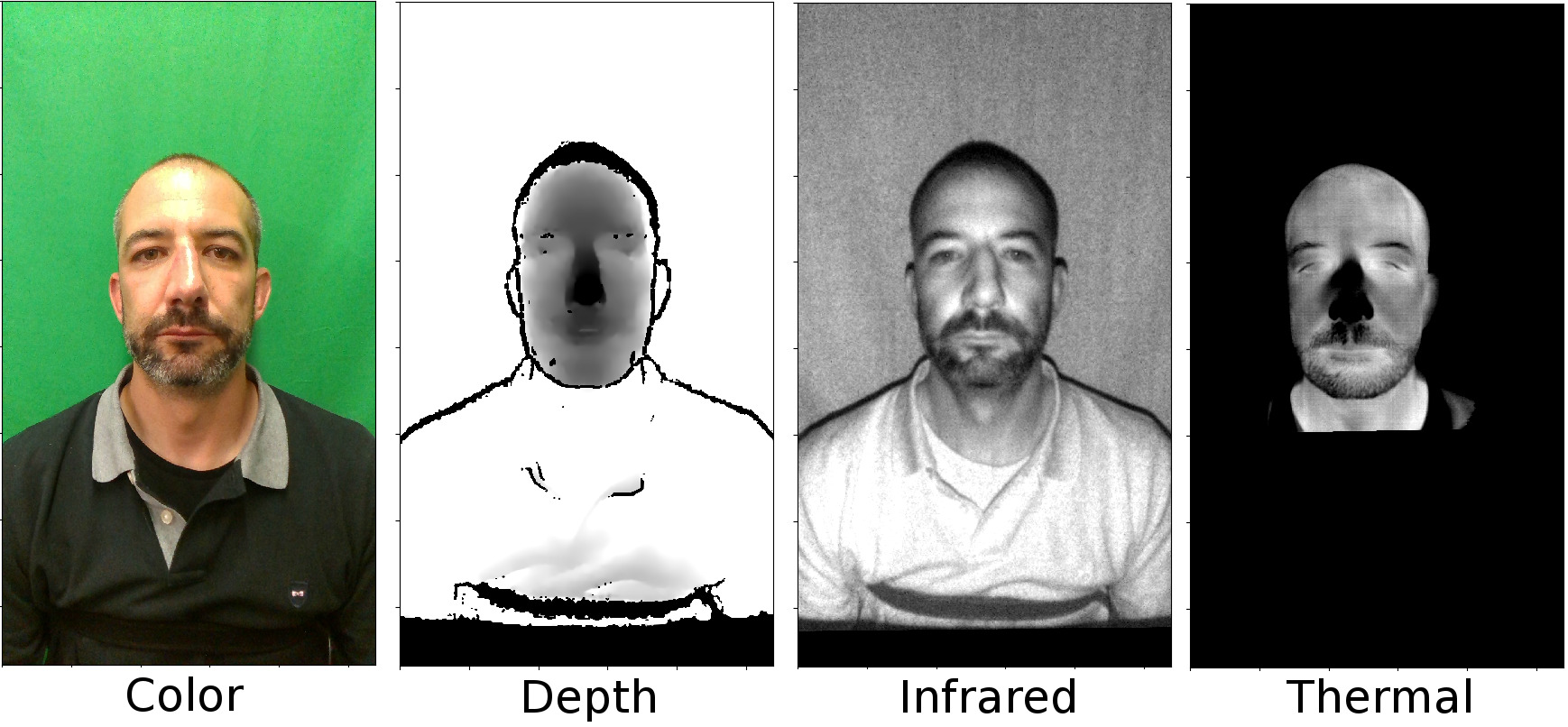
Sample from the WMCA Dataset of bonafide accesses for the four acquisition channels after alignment. The images from all channels are aligned with the calibration parameters and normalized to eight bits for better visualization.
I worked with various different themes in computer vision applied to biometric recognition. In here, I highlight some of the more recent contributions:
- Presentation Attack Detection (PAD): Biometric recognition systems are exposed to presentation attacks, and dectectors for this purpose are required building blocks of thrustworthy systems. Most PAD systems work discriminatively, trying to separate attacks from bona fide presentations. We showed this technique does not generalize well to unseen presentation attacks. We explored, for the first time, alternate approaches by joint-modelling client identity as a way to calibrate PAD output scores [1], showing increased robustness to unseen attacks. More recently, we also showed that solely modelling bona fide presentations is also an effective way to increased PAD robustness [2]. Finally, we showed that by adding heterogenous inputs to PAD systems can improve their robustness [3] to achieve state-of-the-art performance, even to unseen conditions during training.
- Domain Specific Units (Adaptation): In [4], we apply domain adaptation via dedicated Domain-Specific Units (DSU), with an application to Heterogeneous Face Recognition. In this class of problems, one wants to recognize an individual across different spectral data, based on the representation on a principal spectrum (e.g., visual). It is a challenging task because multi-spectral data for covering large populations is rare, which in turn stymies the training of deep convolution-based architectures for this task. We developed a mechanism to adapt the parameters of models pre-trained on large visual spectral face recognition datasets, which are readily available. My contributions are directly related to core idea of this work.
Reproducibility Checklist
- All published papers are 100% reproducible
- Software is open-source, extensible and tested on a regular basis
- Complete documentation is available
- For details on each publication, check the publication directly.
Datasets
Some of the datasets published during this period:
- WMCA "Wide Multi Channel Presentation Attack" Database, DOI: 10.34777/8zdh-v182
- Replay Attack Database, DOI: 10.34777/cwcg-7r82
- COHFACE Database, DOI: 10.34777/ff3f-ba56
Partnership
Work in Biometrics was conducted on the context of various SNSF, EU FP7, IARPA, and Innosuisse projects together with the Biometrics Security & Privacy Group at Idiap.
The ensemble of this work was published as book chapters, international peer-reviewed scientific journals (including articles at very high-impact factor journals) and in peer-reviewed conference papers totalling a few thousand citations.
Bibliography
[1] Ivana Chingovska and André Anjos. On the use of client identity information for face anti-spoofing. IEEE Transactions on Information Forensics and Security, Special Issue on Biometric Anti-spoofing, 10(4):787–796, February 2015. doi:10.1109/TIFS.2015.2400392.
@article{tifs-2015,
author = "Chingovska, Ivana and Anjos, Andr{\'{e}}",
keywords = "Biometric Verification, Counter-Measures, Counter-Spoofing, Liveness Detection, Replay, Spoofing Attacks",
title = "On the use of client identity information for face anti-spoofing",
journal = "IEEE Transactions on Information Forensics and Security, Special Issue on Biometric Anti-spoofing",
addendum = "(Issued from Ph.D co-supervision)",
volume = "10",
number = "4",
month = "February",
year = "2015",
pages = "787--796",
doi = "10.1109/TIFS.2015.2400392",
pdf = "https://www.idiap.ch/\textasciitilde aanjos/papers/tifs-2015.pdf",
abstract = "With biometrics playing the role of a password which can not be replaced if stolen, the necessity of establishing counter-measures to biometric spoofing attacks has been recognized. Regardless of the biometric mode, the typical approach of anti-spoofing systems is to classify biometric evidence based on features discriminating between real accesses and spoofing attacks. For the first time, to the best of our knowledge, this paper studies the amount of client-specific information within these features and how it affects the performance of anti-spoofing systems. We make use of this information to build two client-specific anti-spoofing solutions, one relying on a generative and another one on a discriminative paradigm. The proposed methods, tested on a set of state-of-the-art anti-spoofing features for the face mode, outperform the client-independent approaches with up to 50\\% relative improvement and exhibit better generalization capabilities on unseen types of spoofing attacks."
}
[2] Olegs Nikisins, Amir Mohammadi, André Anjos, and Sébastien Marcel. On effectiveness of anomaly detection approaches against unseen presentation attacks in face anti-spoofing. In The 11th IAPR International Conference on Biometrics (ICB 2018). February 2018. URL: https://publications.idiap.ch/index.php/publications/show/3793, doi:10.1109/ICB2018.2018.00022.
@inproceedings{icb-2018,
author = "Nikisins, Olegs and Mohammadi, Amir and Anjos, André and Marcel, Sébastien",
month = "February",
title = "On Effectiveness of Anomaly Detection Approaches against Unseen Presentation Attacks in Face Anti-Spoofing",
booktitle = "The 11th IAPR International Conference on Biometrics (ICB 2018)",
year = "2018",
url = "https://publications.idiap.ch/index.php/publications/show/3793",
pdf = "https://www.idiap.ch/\textasciitilde aanjos/papers/icb-2018.pdf",
doi = "10.1109/ICB2018.2018.00022",
abstract = "While face recognition systems got a significant boost in terms of recognition performance in recent years, they are known to be vulnerable to presentation attacks. Up to date, most of the research in the field of face anti-spoofing or presentation attack detection was considered as a two-class classification task: features of bona-fide samples versus features coming from spoofing attempts. The main focus has been on boosting the anti-spoofing performance for databases with identical types of attacks across both training and evaluation subsets. However, in realistic applications the types of attacks are likely to be unknown, potentially occupying a broad space in the feature domain. Therefore, a failure to generalize on unseen types of attacks is one of the main potential challenges in existing anti-spoofing approaches. First, to demonstrate the generalization issues of two-class anti-spoofing systems we establish new evaluation protocols for existing publicly available databases. Second, to unite the data collection efforts of various institutions we introduce a challenging Aggregated database composed of 3 publicly available datasets: Replay-Attack, Replay-Mobile and MSU MFSD, reporting the performance on it. Third, considering existing limitations we propose a number of systems approaching a task of presentation attack detection as an anomaly detection, or a one-class classification problem, using only bona-fide features in the training stage. Using less training data, hence requiring less effort in the data collection, the introduced approach demonstrates a better generalization properties against previously unseen types of attacks on the proposed Aggregated database."
}
[3] Anjith George, Zohreh Mostaani, David Geissenbuhler, Olegs Nikisins, André Anjos, and Sébastien Marcel. Biometric face presentation attack detection with multi-channel convolutional neural network. IEEE Transactions on Information Forensics and Security, May 2019. doi:10.1109/TIFS.2019.2916652.
@article{tifs-2019-2,
author = "George, Anjith and Mostaani, Zohreh and Geissenbuhler, David and Nikisins, Olegs and Anjos, Andr{\'{e}} and Marcel, S{\'{e}}bastien",
title = "Biometric Face Presentation Attack Detection with Multi-Channel Convolutional Neural Network",
journal = "IEEE Transactions on Information Forensics and Security",
month = "May",
year = "2019",
doi = "10.1109/TIFS.2019.2916652",
pdf = "https://www.idiap.ch/\textasciitilde aanjos/papers/tifs-2019-2.pdf",
abstract = "Face recognition is a mainstream biometric authentication method. However, vulnerability to presentation attacks (a.k.a spoofing) limits its usability in unsupervised applications. Even though there are many methods available for tackling presentation attacks (PA), most of them fail to detect sophisticated attacks such as silicone masks. As the quality of presentation attack instruments improves over time, achieving reliable PA detection with visual spectra alone remains very challenging. We argue that analysis in multiple channels might help to address this issue. In this context, we propose a multi-channel Convolutional Neural Network based approach for presentation attack detection (PAD). We also introduce the new Wide Multi-Channel presentation Attack (WMCA) database for face PAD which contains a wide variety of 2D and 3D presentation attacks for both impersonation and obfuscation attacks. Data from different channels such as color, depth, near-infrared and thermal are available to advance the research in face PAD. The proposed method was compared with feature-based approaches and found to outperform the baselines achieving an ACER of 0.3\\% on the introduced dataset. The database and the software to reproduce the results are made available publicly."
}
[4] Tiago de Freitas Pereira, André Anjos, and Sébastien Marcel. Heterogeneous face recognition using domain specific units. IEEE Transactions on Information Forensics and Security, December 2019. URL: https://publications.idiap.ch/index.php/publications/show/3963, doi:10.1109/TIFS.2018.2885284.
@article{tifs-2019,
author = "de Freitas Pereira, Tiago and Anjos, André and Marcel, Sébastien",
month = "December",
title = "Heterogeneous Face Recognition Using Domain Specific Units",
journal = "IEEE Transactions on Information Forensics and Security",
year = "2019",
addendum = "(Issued from Ph.D co-supervision)",
doi = "10.1109/TIFS.2018.2885284",
url = "https://publications.idiap.ch/index.php/publications/show/3963",
pdf = "https://www.idiap.ch/\textasciitilde aanjos/papers/ieee-tifs-2018.pdf",
abstract = "The task of Heterogeneous Face Recognition consists in matching face images that are sensed in different domains, such as sketches to photographs (visual spectra images), thermal images to photographs or near-infrared images to photographs. In this work we suggest that high level features of Deep Convolutional Neural Networks trained on visual spectra images are potentially domain independent and can be used to encode faces sensed in different image domains. A generic framework for Heterogeneous Face Recognition is proposed by adapting Deep Convolutional Neural Networks low level features in, so called, “Domain Specific Units”. The adaptation using Domain Specific Units allow the learning of shallow feature detectors specific for each new image domain. Furthermore, it handles its transformation to a generic face space shared between all image domains. Experiments carried out with four different face databases covering three different image domains show substantial improvements, in terms of recognition rate, surpassing the state-of-the-art for most of them. This work is made reproducible: all the source code, scores and trained models of this approach are made publicly available."
}